Fundamental Principles for Leveraging Generative AI in Corporations
- Xiao Zhong
- Feb 19
- 3 min read
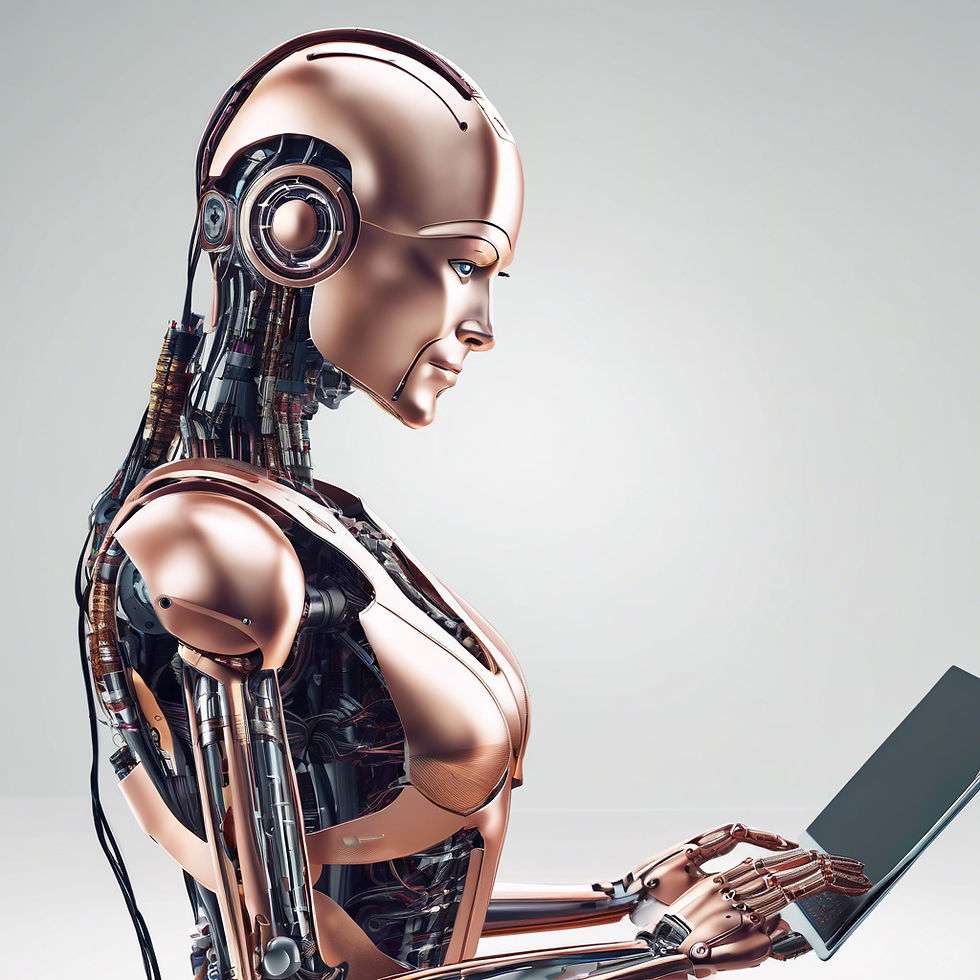
GenAI is transforming industries by offering corporations new ways to innovate, optimize operations, and drive competitive advantages. However, implementing GenAI effectively requires careful strategic planning. While most industries are still catching up with the digital transformation wave, integrating GenAI into their strategies presents additional challenges and opportunities.
Based on numerous discussions with key stakeholders across various industries and AI experts, I am listing the key considerations for corporations looking to integrate Generative AI into their business models.
1. Define Business Objectives and Use Cases
Before adopting GenAI, corporations must clearly define their goals. Common business objectives include:
Enhancing customer experiences (e.g., AI-driven chatbots, personalized recommendations)
Automating internal workflows (e.g., content generation, document summarization, coding assistance)
Improving decision-making (e.g., predictive analytics, AI-powered insights)
Driving innovation (e.g., AI-assisted R&D, generative design)
Aligning GenAI applications with core business objectives ensures that the technology delivers measurable value.
2. Assess Data Readiness and Quality
Similar to the "big data" era started over a decade ago (feels like ages now), genAI models rely heavily on high-quality data. Companies must evaluate:
Data availability: Do they have sufficient structured and unstructured data for training and fine-tuning AI models?
Data integrity and bias: How clean, unbiased, and representative is the data?
Data governance: Are there strong policies in place to ensure compliance with privacy laws like GDPR and CCPA?
A well-defined data strategy is essential for extracting meaningful insights from AI models.
3. Choose Between Off-the-Shelf and Custom AI Solutions
Corporations must decide whether to adopt pre-built GenAI tools or develop custom AI models:
Off-the-shelf solutions (e.g., OpenAI's GPT, Google's Gemini, Anthropic’s Claude) are quick to implement but may lack industry-specific customizations.
Custom-built models allow for greater control and optimization but require significant investment in AI expertise and infrastructure.
A hybrid approach—leveraging foundational models while fine-tuning for specific business needs—often provides the best balance.
4. Evaluate Cost and ROI
Deploying GenAI at scale can be expensive due to computing power, licensing fees, and data storage. Companies should assess:
Total Cost of Ownership (TCO): Costs for cloud hosting, model training, and ongoing maintenance.
Return on Investment (ROI): Projected efficiency gains, revenue growth, and cost savings.
Scalability: Can the AI solution grow alongside the business without excessive costs?
5. Ensure Ethical AI and Compliance
Ethical considerations are paramount in AI adoption. Companies must address:
Bias and fairness: Implement safeguards to prevent discriminatory AI outputs.
Transparency: Ensure AI-driven decisions are explainable and auditable.
Regulatory compliance: Stay updated on AI governance frameworks, such as the EU AI Act or NIST AI Risk Management Framework.
Establishing an AI ethics board and responsible AI guidelines can help mitigate risks.
6. Integrate AI into Workforce Strategy
GenAI adoption impacts workforce dynamics. Companies should:
Identify upskilling opportunities for employees to work effectively with AI.
Redefine job roles to leverage AI as an augmentation tool rather than a replacement.
Foster an AI-driven culture to encourage adoption across teams.
7. Develop a Scalable AI Infrastructure
A solid technology stack is necessary for GenAI success. Key infrastructure components include:
Cloud vs. on-premise computing decisions based on security and scalability needs.
APIs and integrations to connect AI capabilities with existing enterprise systems.
MLOps (Machine Learning Operations) to manage AI lifecycle, model updates, and performance monitoring.
8. Measure Performance and Iterate
Corporations should establish key performance indicators (KPIs) to track GenAI effectiveness, such as:
Accuracy and relevance of AI outputs
Reduction in manual workload and efficiency gains
Customer engagement improvements
Regularly refining AI strategies based on performance data ensures long-term success.
Conclusion
Generative AI holds immense potential for corporations, but its successful implementation requires careful planning. By aligning AI initiatives with business goals, ensuring data readiness, addressing ethical considerations, and fostering an AI-driven workforce, companies can maximize their return on investment and drive meaningful transformation.
If you are passionate about gen AI strategy or are in the process of setting up one for your organization. Drop me a line at xiao@disrupnovation.com. I'd love to connect.
Comentarios